Are you struggling to gather meaningful insights from your audience? Many product owners and UX researchers face the challenge of obtaining accurate data to enhance their products.
Understanding survey sampling is crucial for obtaining reliable information. Picture this: You put in the effort, but the data falls short.
Fret not! This article breaks down survey sampling, making it easy for product owners and UX researchers to create surveys that truly capture the pulse of their audience.
Let's simplify the process and boost the effectiveness of your data collection.
What is survey sampling?
Survey sampling is the method of selecting a subset from a larger population to gather insights and draw conclusions. It helps product owners and UX researchers understand user preferences and behaviors without examining every individual.
By sampling, they can efficiently collect data to make informed decisions about their products. The process involves choosing a representative group that mirrors the diversity of the entire user base.
This ensures that findings accurately reflect the larger population. Survey sampling streamlines research efforts, saving time and resources while providing valuable information to enhance products and user experiences.
Now that we understand the basics of survey sampling, let's explore why it holds a crucial role in the research and decision-making process.
Why is survey sampling important?
Understand the significance of survey sampling in obtaining reliable and representative data. Learn how this process aids in drawing meaningful conclusions, providing a solid foundation for informed decision-making in product development:
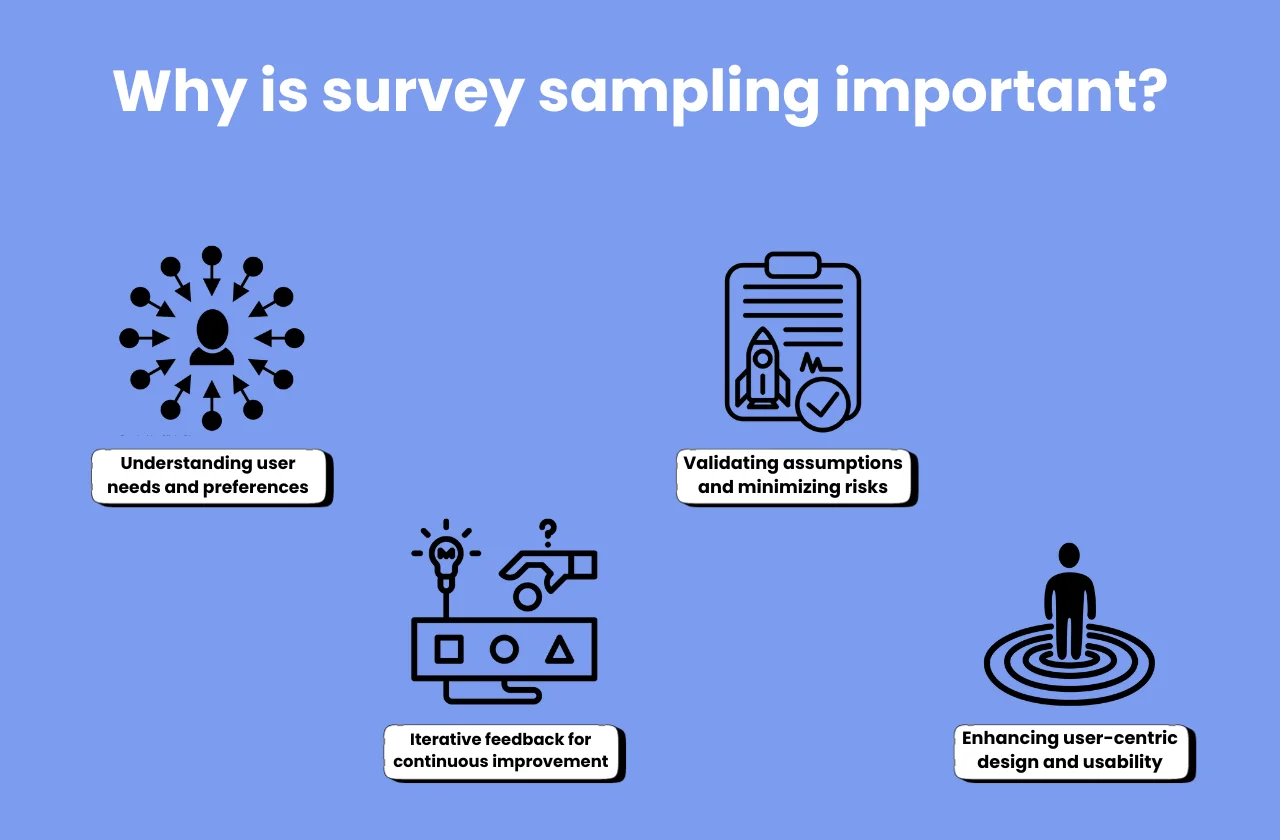
1) Understanding user needs and preferences
One primary advantage of survey sampling is its ability to uncover user needs and preferences.
By reaching out to a representative sample of your target audience, you gain insights into what features resonate with users and what aspects need improvement. This direct input helps in tailoring the product to meet user expectations.
Understanding user preferences is fundamental for enhancing user experience and ensuring that your product aligns with customer expectations from the initial design phases.
2) Iterative feedback for continuous improvement
Survey sampling provides a continuous feedback loop essential for iterative product development.
Regularly engaging with users through surveys allows you to assess the impact of changes or new features, identify areas for improvement, and refine the product accordingly.
This iterative approach ensures that your product evolves in sync with user needs, ultimately enhancing its usability and overall satisfaction.
By incorporating feedback at every stage, you create a product that adapts and resonates with users over time.
3) Validating assumptions and minimizing risks
In the realm of product development, assumptions can pose significant risks. Survey sampling serves as a powerful tool for validating assumptions before committing resources.
By posing targeted questions to your audience, you can confirm or challenge assumptions about user behavior, preferences, and pain points.
This validation process minimizes the risks associated with relying on untested assumptions, guiding your product development efforts with a more evidence-based approach.
4) Enhancing user-centric design and usability
User-centric design is at the core of successful products, and survey sampling plays a pivotal role in achieving this goal.
Through surveys, you gain a deeper understanding of how users interact with your product, allowing you to refine its design for optimal usability.
By identifying pain points and areas of confusion, you can implement design changes that enhance the overall user experience.
Survey sampling ensures that your product remains user-focused, resulting in higher satisfaction and increased chances of success in the market.
Having established why survey sampling is crucial, let's explore the different methods employed in this critical process.
Survey sampling methods
Survey sampling methods fall into two categories: probability sampling and non-probability sampling. Gain insights into the distinct approaches within each category, equipping yourself with the knowledge to choose the most suitable method for your research objectives:
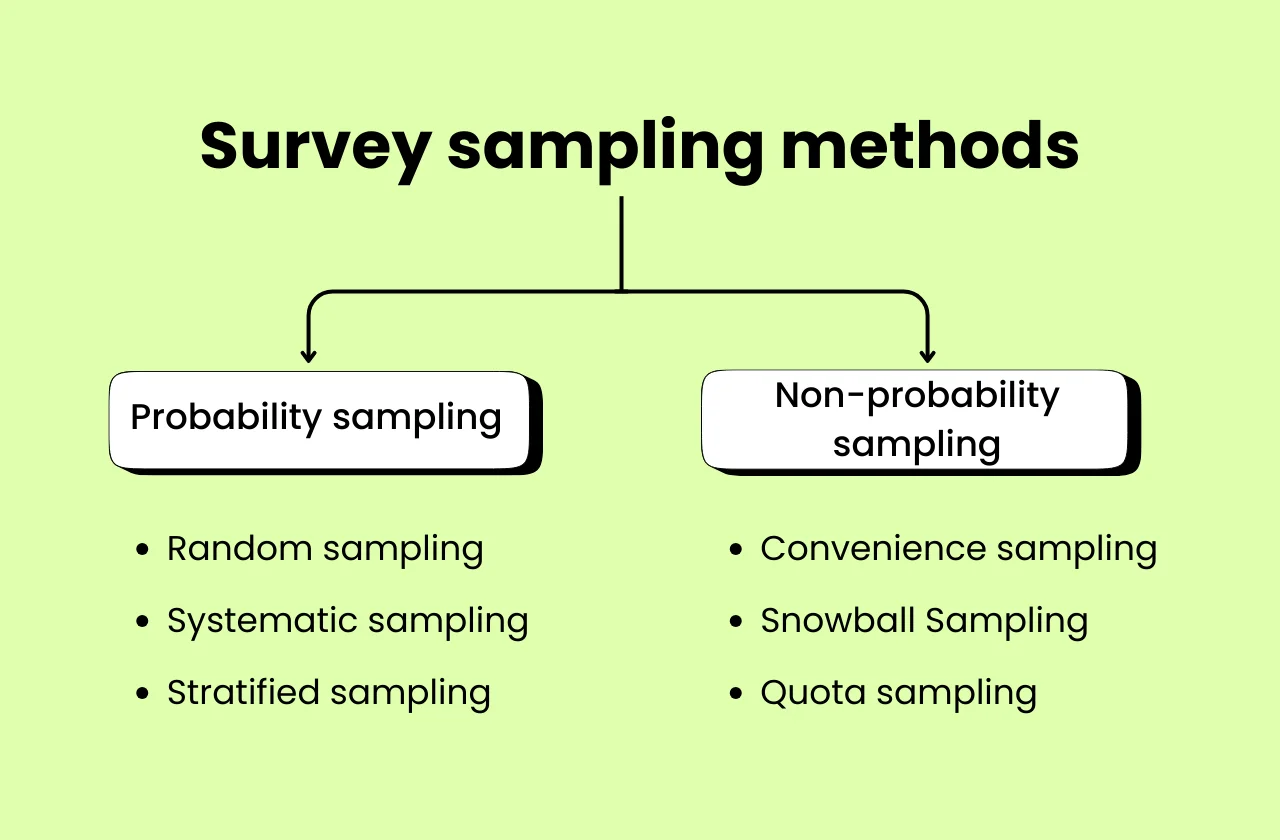
Probability sampling methods:
1) Random sampling
Random sampling stands as the gold standard in survey methodology, ensuring each member of the population has an equal chance of being selected. This method eliminates bias, fostering a representative sample. It is like plucking names from a hat, guaranteeing a diverse and unbiased selection.
Strengths:
- Unbiased representation: Every individual in the population has an equal likelihood of being chosen, ensuring a fair and unbiased representation.
- Statistical inference: Results from random sampling can be generalized to the entire population with a high degree of confidence, making it robust for drawing broader conclusions.
- Simplicity: Its simplicity facilitates ease of implementation, making it a pragmatic choice in many scenarios.
Weaknesses:
- Resource-Intensive: In larger populations, the logistics and costs of conducting a random sample can be demanding, both in terms of time and resources.
- Impracticality in some contexts: In situations where a complete population list is unavailable or impractical, implementing random sampling becomes challenging.
Suitability for different scenarios:
- Ideal for large and diverse populations where a representative sample is crucial.
- Well-suited for studies requiring high generalizability and statistical validity.
2) Systematic sampling
Systematic sampling involves selecting every nth individual from a list after choosing a random starting point. It offers a compromise between simplicity and randomness, providing a structured yet unbiased approach.
Strengths:
- Efficiency: Systematic sampling is more efficient than random sampling, especially in scenarios with a well-organized population list.
- Uniform coverage: This method ensures even coverage across the population, minimizing the risk of overlooking specific groups.
Weaknesses:
- Pattern sensitivity: If there is an underlying pattern in the population list, systematic sampling may introduce bias.
- Sensitivity to starting point: The choice of the initial element can impact the representativeness of the sample.
Suitability for different scenarios:
- Well-suited for large populations where a systematic and organized approach is necessary.
- Effective when a complete population list is available and ordered.
3) Stratified sampling
Stratified sampling involves dividing the population into homogeneous subgroups or strata based on relevant characteristics. Samples are then randomly drawn from each stratum. This method ensures representation from all relevant segments of the population.
Strengths:
- Precision: Provides more precise insights for specific subgroups, allowing for in-depth analysis within defined categories.
- Reduced variability: Minimizes variability within each stratum, enhancing the accuracy of results.
Weaknesses:
- Complexity: Implementation can be more complex than other methods, requiring a thorough understanding of the population's characteristics.
- Need for prior knowledge: Requires prior knowledge about the population to create meaningful strata.
Suitability for different scenarios:
- Effective when the population exhibits significant diversity in relevant characteristics.
- Useful for studies aiming to uncover insights within specific subgroups.
Non-probability sampling methods:
1) Convenience sampling
Convenience sampling involves selecting individuals who are most accessible or convenient for the researcher. This method is pragmatic but lacks the randomness inherent in probability sampling.
Strengths:
- Cost-effective: Convenient and economical, particularly in terms of time and resources.
- Easy implementation: Quick to execute, making it suitable for exploratory or preliminary research.
Weaknesses:
- Bias: Prone to selection bias as it may not represent the broader population accurately.
- Limited generalizability: Results cannot be confidently generalized to the entire population.
Suitability for different scenarios:
- Appropriate for exploratory research or situations where time and resources are limited.
- Often used in the early stages of research to generate initial insights.
2) Snowball Sampling
Snowball sampling involves recruiting participants through existing contacts. Initially, a few participants are identified and then asked to refer to others. This method is particularly useful when the target population is challenging to reach directly.
Strengths:
- Access to hard-to-reach population: Effective in situations where the target population is elusive or lacks a clear sampling frame.
- Cost-effective: Similar to convenience sampling, it can be relatively cost-effective.
Weaknesses:
- Bias: Prone to referral bias, as participants may refer to individuals with similar characteristics.
- Limited generalizability: Similar to convenience sampling, the results may not be broadly applicable.
Suitability for different scenarios:
- Ideal for studying populations that are hard to reach or define.
- Suited for exploratory research where the focus is on depth rather than representativeness.
3) Quota sampling
Quota sampling involves setting specific quotas for certain characteristics and then non-randomly selecting participants to meet these quotas. It aims to ensure proportional representation of key variables.
Strengths:
- Control over sample composition: Enables researchers to control the composition of the sample by setting quotas for relevant variables.
- Simplicity: Easier to implement compared to stratified sampling, providing a balance between control and practicality.
Weaknesses:
- Potential for bias: If quotas are not set appropriately, there is a risk of introducing bias.
- Limited generalizability: Results may not be as broadly applicable as those obtained through probability sampling.
Suitability for different scenarios:
- Suitable when certain key characteristics are crucial, and researchers seek control over sample composition.
- Often used in market research when specific demographic groups need representation.
Now that we've covered the methods, let's explore how survey samples are applied specifically in product development research.
How to use survey sampling in product development research
Explore how survey samples play a pivotal role in product development research. Discover the practical applications of sampling methods in gathering user feedback, optimizing user experiences, and enhancing product features based on real user insights:

Step 1: Identifying the target population
Defining the target audience for a survey is pivotal in extracting meaningful insights for product development. It's essential to clearly delineate who the intended users are and their relevant characteristics.
Demographics: Begin by outlining the demographic details of your target population. Identify key factors such as age, gender, location, and occupation that are pertinent to your product's user base. For instance, if developing a fitness app, understanding the age group and fitness level of potential users is crucial.
Behavioral segmentation: Complement demographic data with behavioral segmentation. Recognize patterns in user behavior that align with your product goals. If working on a social media platform, segment users based on frequency of usage, content preferences, and interaction habits.
Accessing and segmenting: Employ a mix of methods to reach your audience. Utilize in-product surveys, social media platforms, email lists, or community forums. Segment your population based on predefined criteria to ensure a representative sample.
Step 2: Choosing the right sampling method
Selecting an appropriate sampling method is integral to the success of your survey. The method chosen should align with your research objectives, available resources, and the characteristics of your target audience.
Random sampling: While resource-intensive, random sampling ensures each member of the target population has an equal chance of being included. This method reduces selection bias, providing a more accurate representation of the user base.
Stratified sampling: Stratify your population into subgroups based on key characteristics. This method is effective when certain characteristics significantly impact user behavior. For example, if developing a language learning app, stratify by proficiency levels to tailor features accordingly.
Trade-offs: Understand the trade-offs associated with each method. Random sampling might be resource-intensive, while convenience sampling may lead to selection bias. Evaluate the pros and cons based on your research goals and available resources.
Step 3: Managing sampling bias
Sampling bias can compromise the validity of your survey results. It's imperative to recognize and mitigate various types of bias to ensure the reliability of your findings.
Selection bias: Address bias introduced during participant selection. Use random selection methods to minimize this bias. Ensure that participants are chosen without any predisposition to skew results.
Response bias: Acknowledge the potential for response bias where participants may provide socially desirable answers. Mitigate this by framing questions neutrally and ensuring anonymity in responses. Pilot testing can identify and rectify wording issues.
Mitigation strategies: Employ strategies like clear question wording, random selection, and pilot testing to minimize bias. Regularly assess and refine your survey instruments to adapt to changing user dynamics.
Step 4: Analyzing survey data
Effectively analyzing survey data is essential for extracting actionable insights. Employ a combination of traditional demographic analysis and leverage modern technologies like AI and ML for a comprehensive understanding.
Demographic analysis: Begin with a thorough analysis of demographic data. Understand the composition of your sample across various parameters. This insight aids in tailoring your product to the specific needs and preferences of your user base.
Key findings exploration: Dive deep into key findings. Identify patterns, correlations, and outliers that can guide product development. For example, if users from a specific demographic show a higher engagement with a particular feature, prioritize its enhancement.
AI and ML integration: Leverage AI and ML tools for in-depth analysis. They can unveil hidden patterns in large datasets, predicting user behavior and preferences. Implement algorithms to automate the identification of trends, allowing for more efficient decision-making.
As we navigate through the practical aspects, it's crucial to be aware of common mistakes and pitfalls associated with survey sampling. Let's address these issues in the next section.
Survey sampling: Common mistakes/pitfalls (and how to avoid them)
Avoid common pitfalls in survey sampling by understanding the challenges that product owners and UX researchers may encounter. We'll provide practical tips and strategies to navigate these pitfalls, ensuring your survey samples yield reliable and actionable insights:
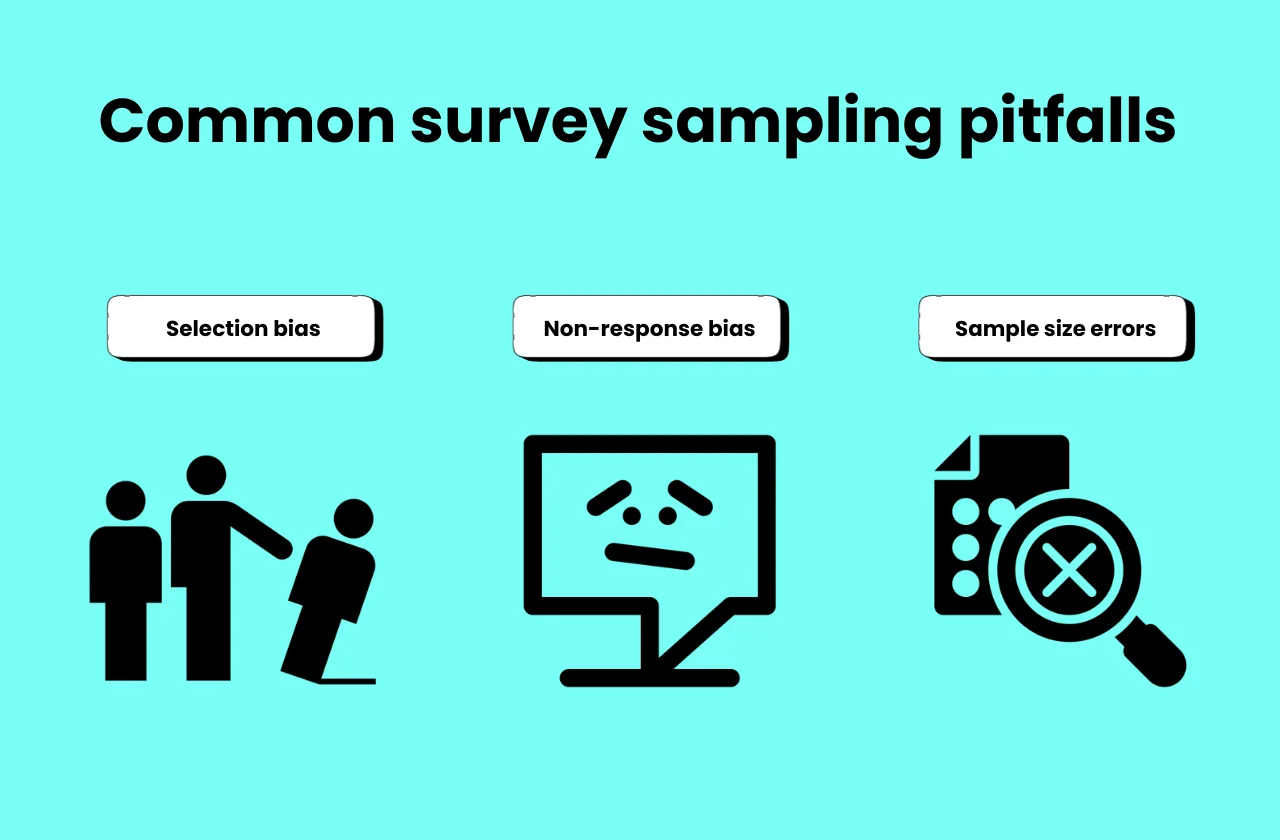
1) Selection bias:
Selection bias, a common stumbling block in survey sampling, occurs when certain individuals have a higher probability of being included in the sample than others.
This distortion can manifest in various sampling methods, like convenience sampling or voluntary response sampling. The impact is profound, skewing research results and compromising the validity of findings.
For instance, if a survey on a new app feature is distributed only to frequent users, it may not capture the sentiments of occasional users or those who have yet to engage with the feature.
This skewed representation can lead to misguided conclusions, affecting the product decisions based on the survey outcomes.
To mitigate selection bias, employ random sampling techniques. This ensures that each member of the population has an equal chance of being included in the sample, providing a more accurate reflection of diverse perspectives.
Random sampling can be achieved through methods like simple random sampling or stratified random sampling, promoting unbiased and representative results.
2) Non-response bias:
Non-response bias arises when individuals chosen for the survey fail to participate, introducing a potential source of distortion. This issue can significantly impact the validity of collected data, as the non-responders may differ systematically from those who participate.
For product owners and UX researchers, ensuring a representative and engaged sample is crucial for actionable insights. To minimize non-response bias, strategic measures are essential.
Firstly, understanding the reasons behind non-response is key. Implement follow-up communication to encourage participation and address concerns that may deter respondents.
Keep the survey concise and engaging, respecting the time constraints of participants. By using reminders and incentives, such as discounts or early access to new features, you can enhance response rates and diminish the risk of non-response bias.
Further, consider employing techniques like weighting to adjust for potential biases introduced by non-response. Weighting assigns different weights to respondents based on their characteristics, compensating for the underrepresentation of specific groups.
This ensures that the findings accurately reflect the broader user base and enables more informed product decisions.
3) Sample size errors:
Incorrect sample size calculations can be a stumbling block in survey sampling, leading to inaccurate results and, consequently, flawed decisions. The significance of an adequate sample size cannot be overstated for product owners and UX researchers seeking robust insights.
Inadequate samples may fail to capture the variability within the population, jeopardizing the reliability of survey outcomes. Thus, avoiding sample size errors requires a meticulous approach.
Begin by clearly defining the desired level of precision and confidence in the survey results. Precision reflects the margin of error tolerated, while confidence represents the level of certainty in the findings. Utilize statistical formulas or online calculators to determine the optimal sample size based on these parameters.
Understand the population variability – the more diverse the population, the larger the sample required. Strive for a balance between precision and practicality. While larger samples enhance precision, they also increase costs and time.
Regularly reassess the sample size calculations throughout the research process, especially if there are unexpected shifts in the population characteristics. This proactive approach ensures that the survey results remain accurate and actionable, guiding product decisions effectively.
Conclusion
In conclusion, survey sampling is a crucial method for product owners and UX researchers to gather valuable insights from a subset of their target audience. By selecting a representative group, they can make informed decisions that enhance product development and user experience.
Understanding the basics, such as random sampling and stratification, empowers professionals to collect reliable data. The simplicity of survey sampling lies in its practicality—efficiently capturing opinions without exhausting resources.
As product owners and UX researchers delve into this straightforward yet powerful approach, they unlock the potential to refine their strategies and create products that resonate with their audience.