Sampling errors plague research, undermining its reliability. They breed doubt in findings, perplexing product owners and UX researchers alike. Without clarity on definitions, types, and examples, pitfalls lurk in every study.
This article elucidates sampling errors, equipping practitioners to identify and mitigate them effectively. Through concrete instances and straightforward explanations, it unveils the nuances of sampling errors.
Understanding these intricacies empowers professionals to fortify their research endeavors, ensuring robust insights and informed decision-making.
In product development and user experience analysis, confronting sampling errors head-on is paramount. Let's delve into the essence of these errors and navigate toward more dependable research outcomes.
What is a sampling error?
Sampling error occurs when the data collected from a subset of a population diverges from the true characteristics of the entire group.
This statistical glitch stems from the reliance on a sample rather than a complete dataset. In the realm of research, it is crucial for product owners and UX researchers to recognize that sampling error can distort findings, leading to inaccurate insights.
The active mitigation of this error involves employing proper sampling techniques, ensuring representative samples, and acknowledging the potential impact on decision-making. Awareness of sampling error is paramount for those navigating the intricacies of data interpretation in product development and user experience research.
Now that we've clarified what sampling errors entail, let's delve into the distinctions between sampling errors and non-sampling errors.
What’s the difference between sampling error and non-sampling error?
Sampling error and non-sampling error are two types of errors that occur in research studies, particularly in sampling methodologies. Understanding their differences is crucial for product owners and UX researchers to ensure accurate data collection and analysis:
Having differentiated between sampling and non-sampling errors, let's explore the various types of sampling methods and the errors associated with them.
Types of sampling and errors
Different sampling methods like random sampling, stratified sampling, and cluster sampling come with their own set of errors. Identifying these methods and errors aids researchers and product owners in making informed decisions about data collection strategies:
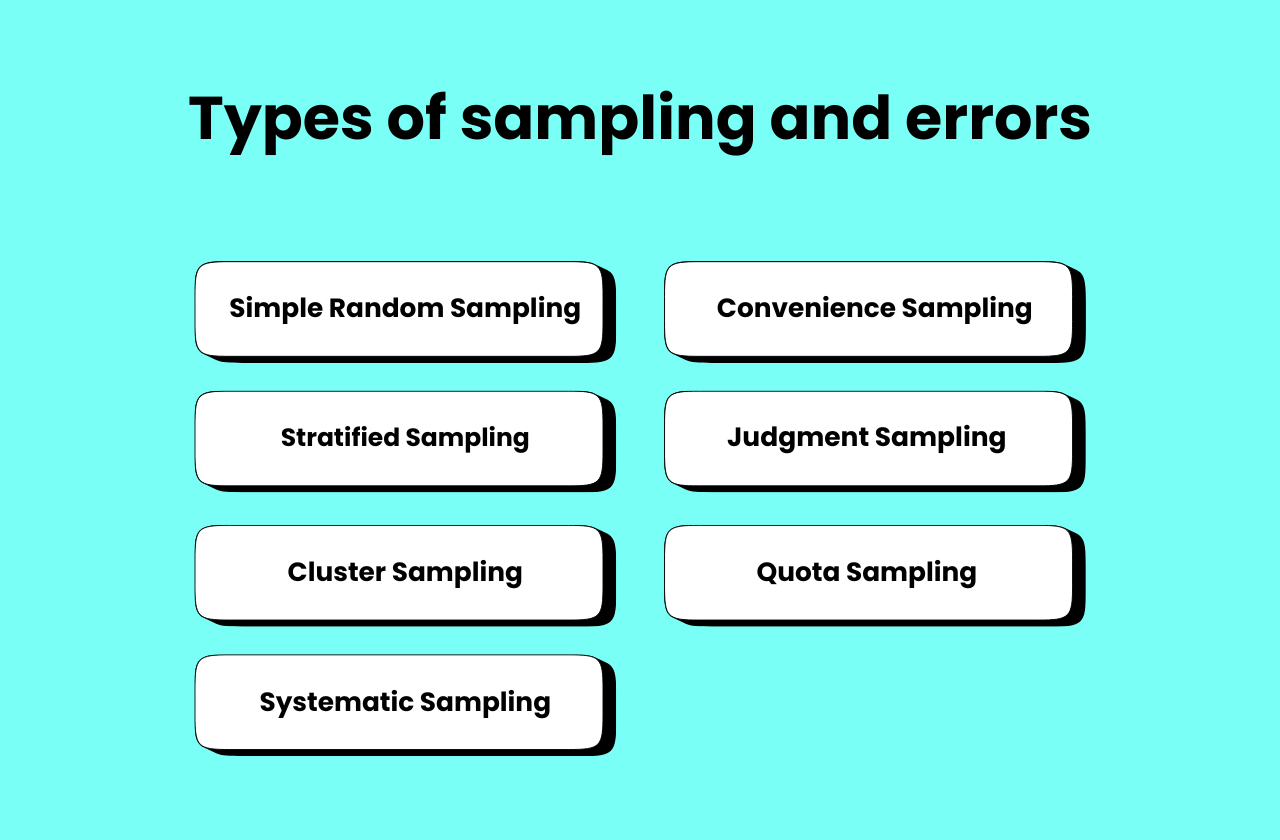
Probability Sampling
Probability sampling methods involve selecting samples based on the principles of probability, ensuring every element in the population has an equal chance of being chosen.
1) Simple Random Sampling
Simple Random Sampling entails selecting a subset of participants from a larger population entirely by chance, ensuring each member has an equal probability of being chosen. Errors associated with this method primarily stem from incomplete sampling frames or biased selection processes.
For instance, in an online survey for a social media app's new feature, if the sampling frame excludes certain user demographics or if users opt out due to survey fatigue, the sample may not accurately represent the entire user base, leading to skewed insights.
2) Stratified Sampling
Stratified Sampling involves dividing the population into distinct subgroups or strata based on relevant characteristics and then selecting samples from each stratum. Errors can arise if the chosen strata are not reflective of the population diversity.
For instance, in testing a new e-commerce website, if the strata fail to represent varied user behaviors or preferences, the sample may not accurately capture the website's usability across different customer segments.
3) Cluster Sampling
Cluster Sampling involves dividing the population into clusters, then randomly selecting clusters and including all members within the chosen clusters in the sample. Errors may occur if clusters are not homogeneous or if there is a lack of variability within clusters.
For example, in testing a mobile gaming app, if clusters are based on geographical regions and fail to consider diverse player demographics within each region, the sample may not effectively represent the app's user base, leading to biased insights.
4) Systematic Sampling
Systematic Sampling involves selecting every nth element from the population after randomly determining the starting point. Errors can arise if there is a pattern or periodicity in the population that aligns with the sampling interval.
For instance, in analyzing user behavior data for a music streaming platform, if users tend to engage more during specific time intervals or days of the week, systematic sampling may inadvertently capture biased snapshots of user interactions, impacting the validity of insights derived.
Non-probability Sampling
Non-probability sampling methods do not rely on random selection principles, making it challenging to generalize findings to the entire population.
1) Convenience Sampling
Convenience Sampling involves selecting participants based on their easy availability or accessibility. Errors can occur due to sample bias, as participants may not represent the broader population.
For instance, in gathering feedback for a mobile banking app, relying solely on app store reviews may exclude users who do not actively engage in providing feedback, leading to skewed perceptions of user satisfaction.
2) Judgment Sampling
Judgment Sampling involves selecting participants based on the researcher's discretion or judgment of their relevance to the study.
Errors may arise if the researcher's judgments are influenced by personal biases or limited understanding of the population characteristics, potentially overlooking valuable insights from underrepresented groups.
3) Quota Sampling
Quota Sampling involves selecting participants based on predetermined quotas to ensure a proportional representation of key population characteristics.
Errors can occur if quotas are not accurately defined or if researchers inadvertently overlook certain demographic groups, leading to incomplete or biased samples that undermine the reliability of research findings.
Now that we understand the different types of sampling methods and associated errors, let's discuss how one can calculate and assess the sampling error.
How do you find the sampling error?
Calculating the sampling error involves statistical techniques that assess the accuracy of the sample in representing the population. This process is integral to ensuring the reliability and validity of research findings:
Step 1: Calculate Standard Error:
To find the sampling error, start by calculating the standard error. Take your population's standard deviation and divide it by the square root of your sample size. For instance, if your population standard deviation is 10 and your sample size is 100, you'd divide 10 by the square root of 100, which is 10.
Step 2: Apply Z-score:
Next, multiply the result you obtained by the Z-score value corresponding to your chosen confidence level (CL). For example, if you want a 95% confidence level, the Z-score is approximately 1.96. So, if your standard error is 1, you'd multiply 1 by 1.96.
Step 3: Calculate Margin of Error:
The product of the standard error and the Z-score is your margin of error. This figure indicates the precision of your sample estimate around the population parameter. In our example, if you multiply 1 by 1.96, your margin of error would be 1.96.
Step 4: Interpretation:
For instance, if you conducted a survey with a sample mean of 50 and a margin of error of 1.96, you can be 95% confident that the true population mean falls between 48.04 and 51.96. This information aids product owners and UX researchers in making informed decisions based on data accuracy and confidence levels.
With a grasp of how sampling errors are calculated, let's examine some real-world examples to better understand their implications.
Examples of sampling errors
By examining concrete examples, we can better understand how sampling errors can be manifested in research and product development. These examples provide practical insights into recognizing and addressing sampling errors effectively:
1) Surveying only early adopters to understand general product sentiment (bias)
Surveying only early adopters can introduce bias in understanding product sentiment. For instance, if a company launches a new app and surveys only users who downloaded it within the first week, they might get skewed feedback.
Early adopters often have different expectations and tolerance levels compared to mainstream users. Consequently, their feedback may not accurately represent the sentiments of the broader user base, leading to decisions based on incomplete or misleading information.

2) Conducting A/B tests with imbalanced user groups (inaccurate results)
When conducting A/B tests with imbalanced user groups, the results can be inaccurate. For example, if an e-commerce platform tests a new checkout process but allocates significantly more users to one variation over the other, it may not reflect genuine user preferences.
The imbalance can distort the outcomes, making it challenging to determine the actual effectiveness of each variation. This can lead to erroneous conclusions and misguided optimization efforts based on flawed data.
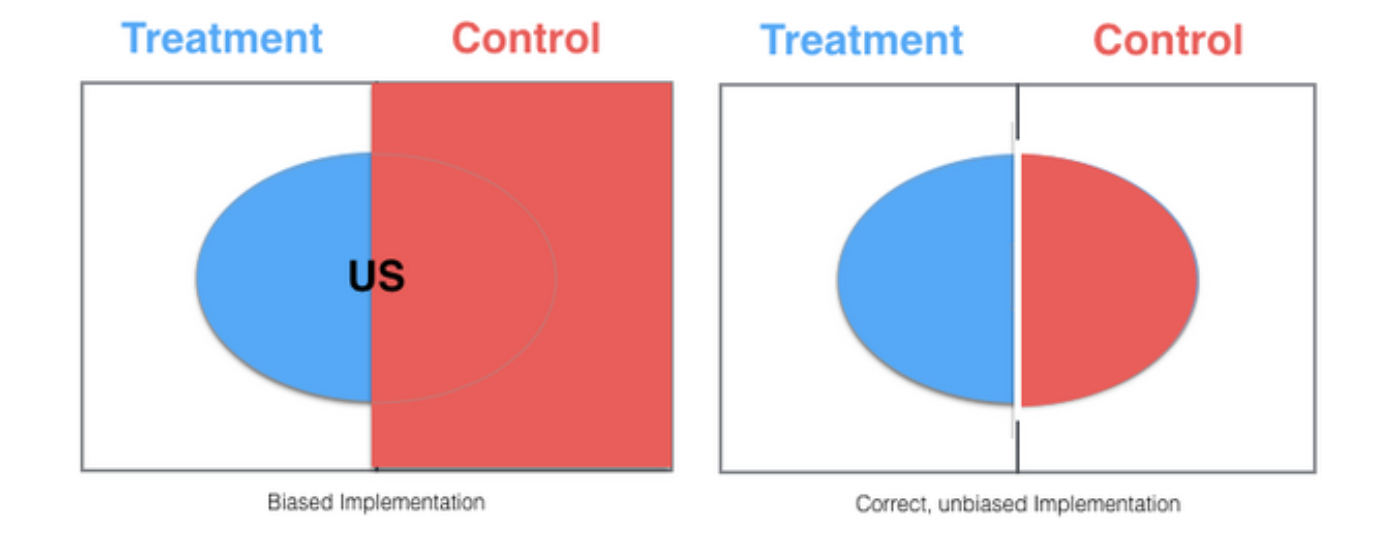
3) Recruiting participants from a single source (lack of diversity)
Recruiting participants from a single source can lead to a lack of diversity in research samples. For instance, if a UX researcher solely relies on recruiting participants from a specific online forum, they may overlook valuable insights from other demographic groups or user segments.
This limited pool of participants can result in a narrow perspective, neglecting the varied needs and preferences of potential users. Consequently, the research findings may not accurately reflect the broader user population, hindering the development of inclusive and user-centric products.
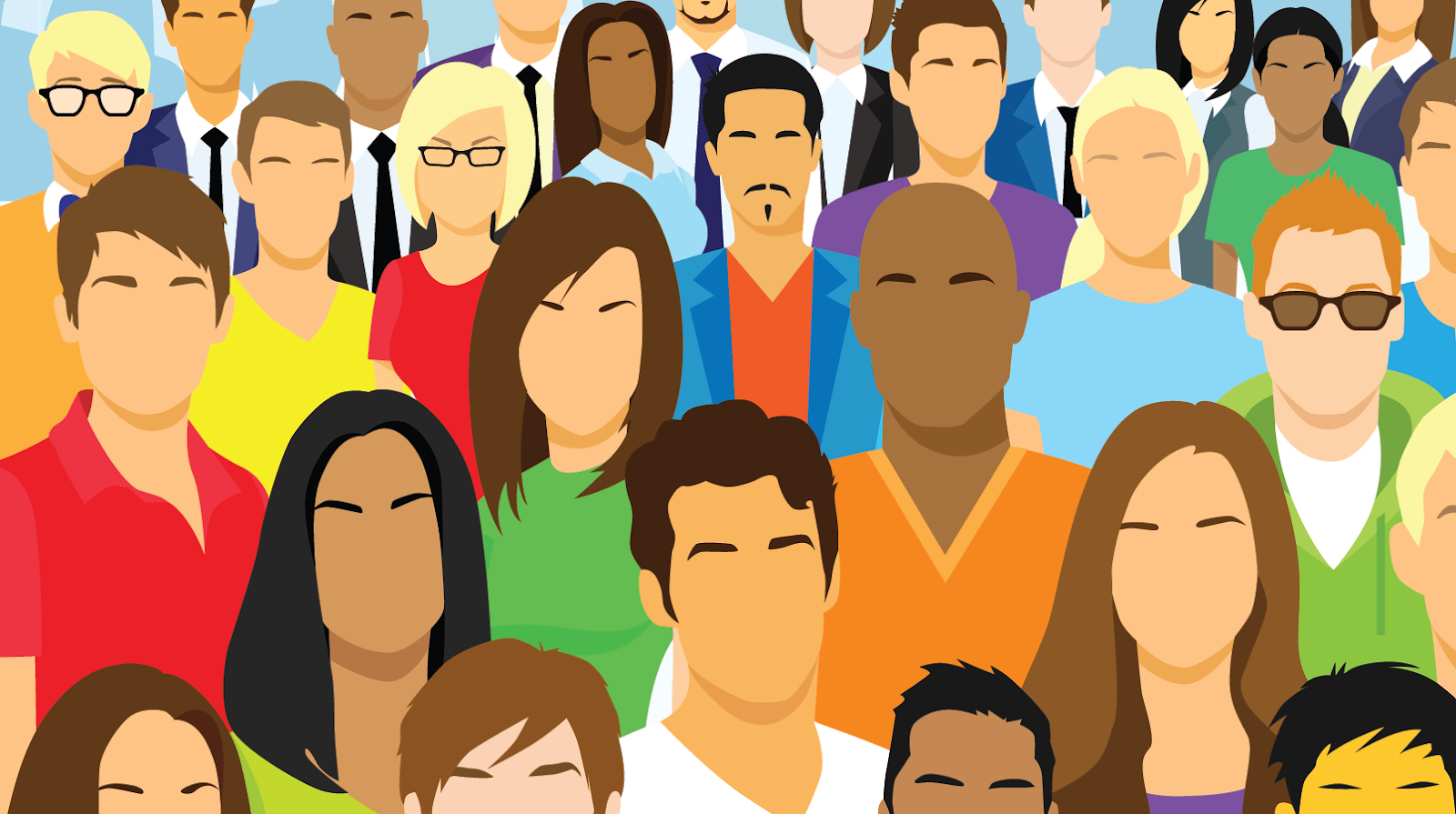
4) Using convenience sampling for usability testing (unrepresentative sample)
Using convenience sampling for usability testing can result in an unrepresentative sample. For example, if a software company recruits usability test participants from its employee pool, it may not capture the diverse range of end-users' experiences and behaviors.
Convenience sampling often entails selecting participants based on ease of access rather than representing the target user population. Consequently, the insights gained from such testing may not generalize well to the intended user base, leading to design decisions that overlook critical user needs and preferences.
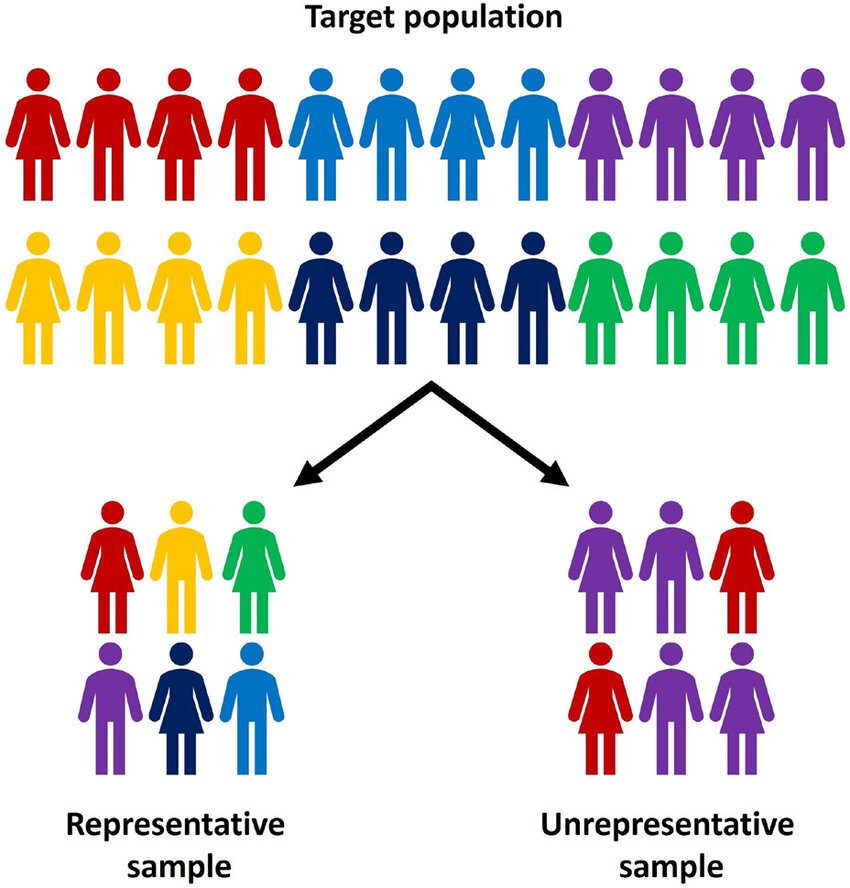
Having explored examples of sampling errors, let's now discuss strategies to minimize these errors and enhance the quality of research outcomes.
How to minimize sampling errors?
Minimizing sampling errors requires meticulous planning, careful execution of sampling methods, and thorough data analysis techniques. Implementing strategies to mitigate these errors enhances the reliability and validity of research findings, empowering product owners and UX researchers in making informed decisions.
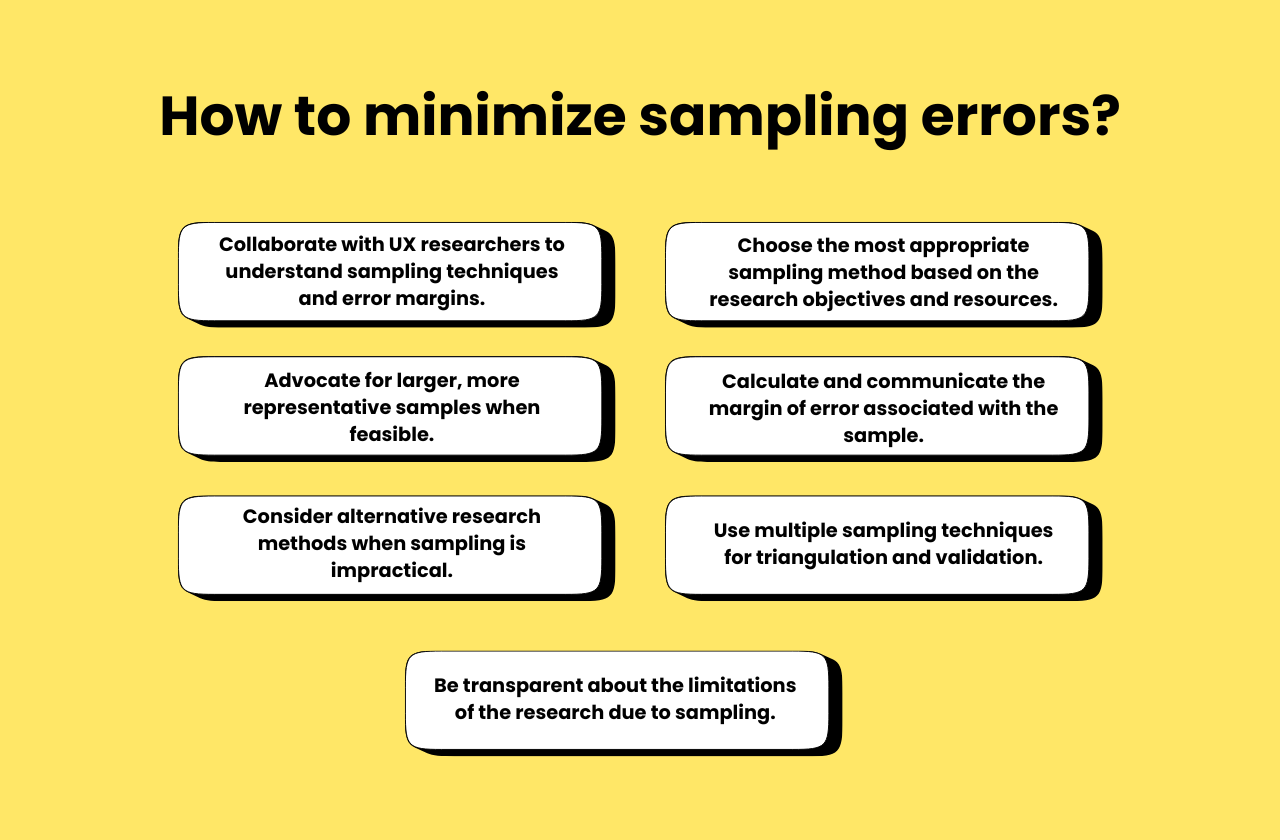
This section provides actionable steps to minimize sampling errors and optimize the research process:
1) Collaborate with UX researchers to understand sampling techniques and error margins.
To minimize sampling errors, collaborate closely with UX researchers. Understand various sampling techniques and error margins.
For instance, if you're conducting a user study on a new mobile app feature, ensure the sample includes diverse user demographics. Collaborating with UX researchers allows for a comprehensive grasp of sampling methodologies and helps tailor research approaches to specific project needs.
2) Advocate for larger, more representative samples when feasible.
Advocate for larger and more representative samples whenever possible. For example, when testing a new website layout, aim to recruit participants from different age groups, backgrounds, and technological proficiencies.
A larger sample size increases the likelihood of capturing diverse perspectives and reduces the margin of error. Advocate for resources and time allocation to achieve representative samples, enhancing the validity and reliability of research findings.
3) Consider alternative research methods when sampling is impractical.
When traditional sampling methods prove impractical, consider alternative research approaches. For instance, if accessing a specific user group is challenging, employ remote usability testing tools to gather feedback from a wider audience.
Utilize online surveys or diary studies to collect data from participants who are geographically dispersed. Exploring alternative methods ensures data collection even under challenging circumstances, reducing the risk of biased or incomplete findings.
4) Choose the most appropriate sampling method based on the research objectives and resources.
Select the most suitable sampling method based on research objectives and available resources. For instance, if investigating user preferences for a new product feature, employ random sampling to ensure every user has an equal chance of participation.
Conversely, purposive sampling may be appropriate when targeting specific user segments with unique characteristics. Tailoring sampling methods to research goals enhances data accuracy and relevance to the project.
5) Calculate and communicate the margin of error associated with the sample.
Calculate and communicate the margin of error associated with the sample to stakeholders. For example, if conducting a survey to gauge customer satisfaction, provide confidence intervals to indicate the range within which the true population parameter lies.
Communicating the margin of error fosters transparency and enables stakeholders to interpret research findings effectively. It also underscores the inherent uncertainty in sampling processes, promoting informed decision-making.
6) Use multiple sampling techniques for triangulation and validation.
Employ multiple sampling techniques to triangulate data and validate research findings. For instance, complement quantitative surveys with qualitative interviews to gain deeper insights into user behavior and preferences.
Conducting user testing in controlled lab environments alongside field observations ensures a comprehensive understanding of user interactions. Integrating diverse sampling methods strengthens the validity and reliability of research outcomes, mitigating the risk of biased interpretations.
7) Be transparent about the limitations of the research due to sampling.
Transparently communicate the limitations of research attributable to sampling constraints. For example, acknowledge any biases inherent in the sample composition or limitations in sample size. When presenting findings, articulate the potential impact of sampling errors on the generalizability of results.
Being transparent about research limitations cultivates trust among stakeholders and encourages critical appraisal of findings. It also prompts discussions on methodological improvements for future research endeavors.
Conclusion
In conclusion, minimizing sampling errors requires collaboration, strategic decision-making, and transparency throughout the research process. By understanding sampling techniques, advocating for representative samples, and employing diverse methods, product owners and UX researchers can enhance the validity and reliability of research outcomes, ultimately informing more informed decision-making and product development.